The landscape of online search is rapidly evolving, thanks to the advancements in Generative AI. Google’s Search Generative Experience (SGE) is at the forefront of this transformation, offering a glimpse into the future of search technology. In this article, we will explore how SGE works, with a specific focus on retrieval-augmented generation, and assess its potential impact on SEO, PPC, and the broader digital marketing ecosystem.
Understanding Search Generative Experience
Search Generative Experience (SGE) is a groundbreaking development in the world of online search. Spearheaded by Google, SGE leverages generative AI to deliver more accurate and context-aware search results. Sundar Pichai’s commitment to the future of SGE highlights its significance in the digital realm.
SGE brings a dramatic shift in how information is processed and surfaced. It poses both opportunities and challenges for businesses that rely on search, both paid and organic, as it can potentially alter the search demand curve and click-through rate (CTR) models.
The Confluence of Generative AI and Information Retrieval
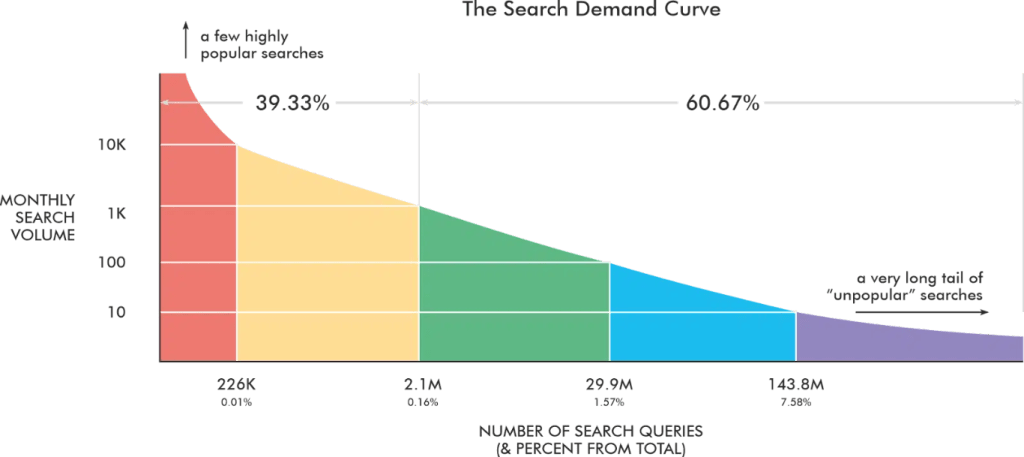
Generative AI, underpinned by natural language processing (NLP), has given rise to large language models (LLMs). These LLMs, based on transformer architectures, have the capacity to generate responses to queries using information extracted from search results. This process is the cornerstone of retrieval-augmented generation (RAG).
Read also: Improving search engine rankings in 2023, 6 important tips!
Retrieval-augmented generation involves incorporating relevant documents or data points, collected based on a query, into the language model’s prompt. This integration “grounds” the model in factual information, minimizing the risk of generating inaccurate responses or hallucinations.
The Birth of RAG
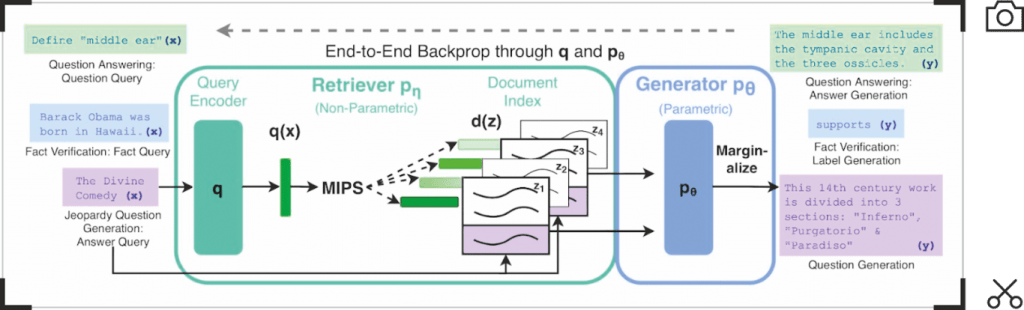
RAG, although popularized by Microsoft’s Bing, was first introduced by the Facebook AI Research team in 2020. Neeva was among the pioneers to implement this paradigm in a public search engine, employing it to power highly specific featured snippets.
RAG is a game-changer because it overcomes the “information-locked” nature of LLMs. It allows these models to consider new information when generating responses, a process similar to Bing’s functionality or real-time crawling with plugins like AIPRM.
Components of RAG
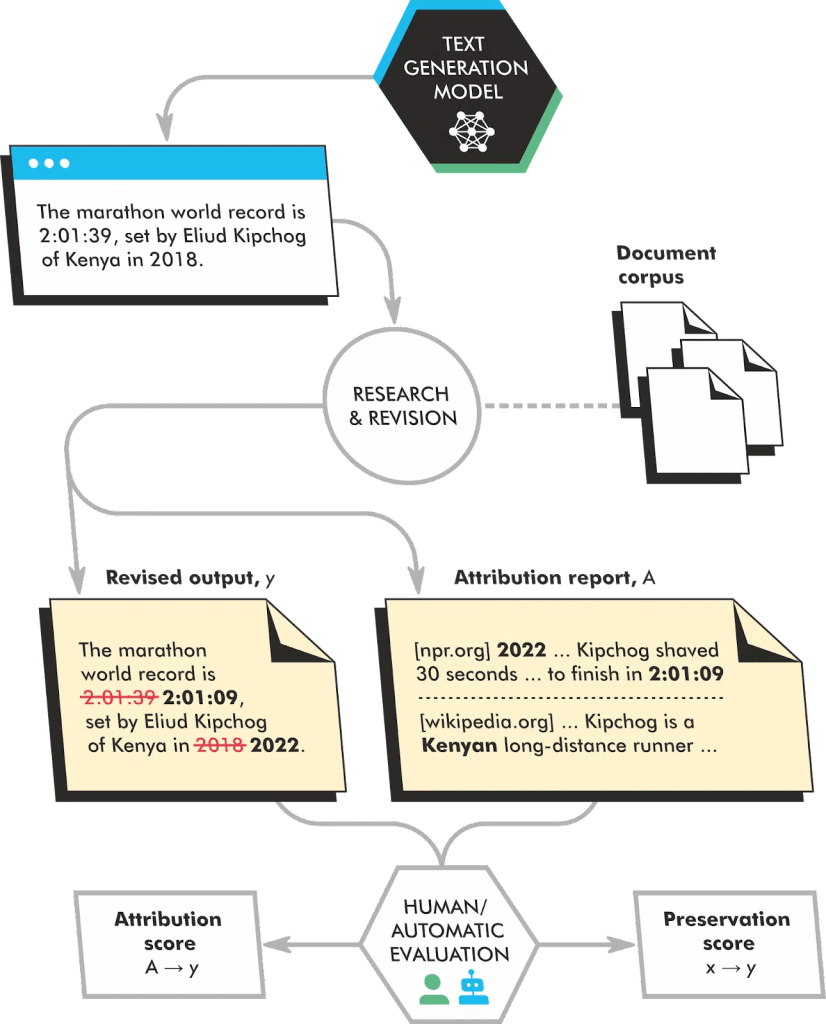
A typical RAG implementation comprises three main components:
- Input Encoder: This component encodes the input prompt into a series of vector embeddings for downstream operations.
- Neural Retriever: It retrieves the most relevant documents from an external knowledge base based on the encoded input prompt.
- Output Generator: This component generates the final output text, considering both the input prompt and the retrieved documents. Pre-trained transformers, like ChatGPT, are commonly used for this purpose.
The synergy between Knowledge Graphs and LLMs
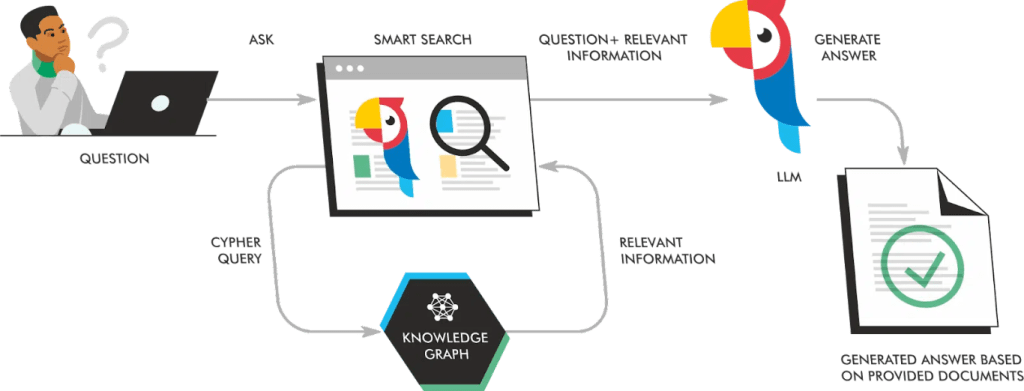
RAG is often implemented using knowledge graphs (KGs) or documents in a vector index. KGs are particularly effective as they provide concise factual data. The integration of KGs with LLMs unleashes the potential of both technologies.
Challenges of RAG
While RAG offers remarkable benefits, there are potential pitfalls to consider:
- Retrieval Quality: The accuracy of the retrieval process is crucial. Inaccurate or irrelevant document retrieval can lead to subpar output.
- Data Quality: RAG’s effectiveness depends on the quality and recency of the data it accesses. Outdated or insufficient data limits its capabilities.
- Redundancy: If retrieved documents overlap substantially, the generated content may become redundant, affecting the quality of results.
- Prompt Length Limits: Prompts can only be so long, which can be limiting when dealing with complex queries.
- Hallucination: Despite rigorous retrieval efforts, LLMs may still produce inaccurate or hallucinatory responses.
Google’s Implementation of RAG in SGE
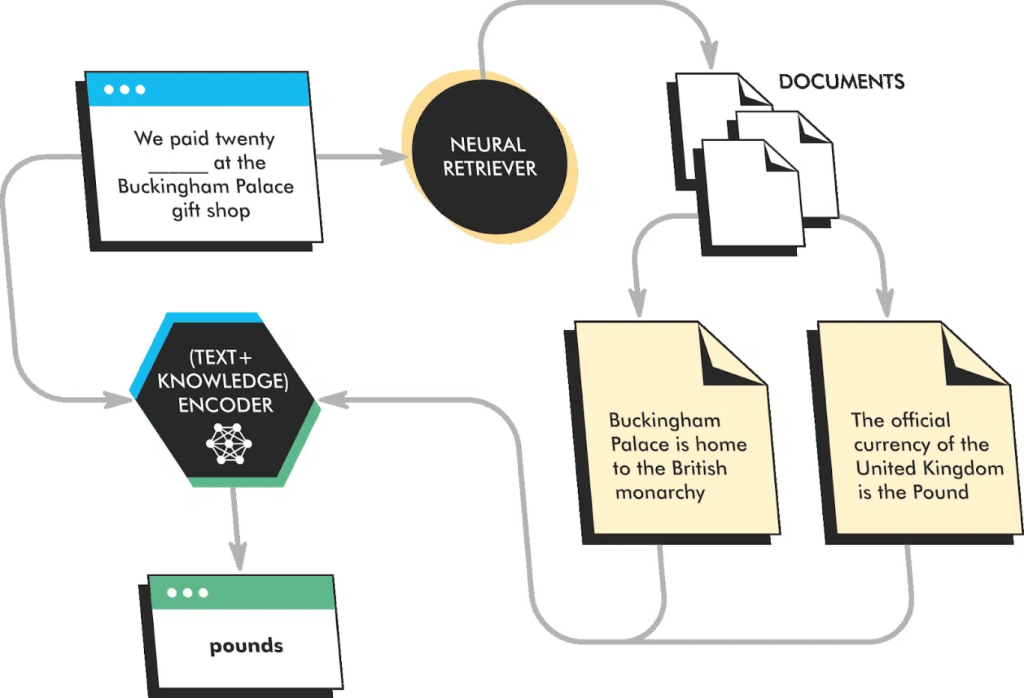
Google has integrated RAG into its Search Generative Experience using a combination of PaLM 2 and MuM language models. The flexibility of Google’s document index and Knowledge Vault allows them to fine-tune responses for better user experiences.
The Evolution of Search Behavior
SGE is set to redefine search behavior in several ways:
- Query Understanding: SGE enhances Google’s ability to understand complex queries, influencing the way users frame their searches.
- The AI Snapshot: The AI snapshot introduces a more comprehensive form of featured snippets, offering generative text and citations.
- Follow-up Questions: Contextual relevance created by preceding results and queries narrows the consideration set of pages, impacting the search journey.
The Threat and Promise of SGE
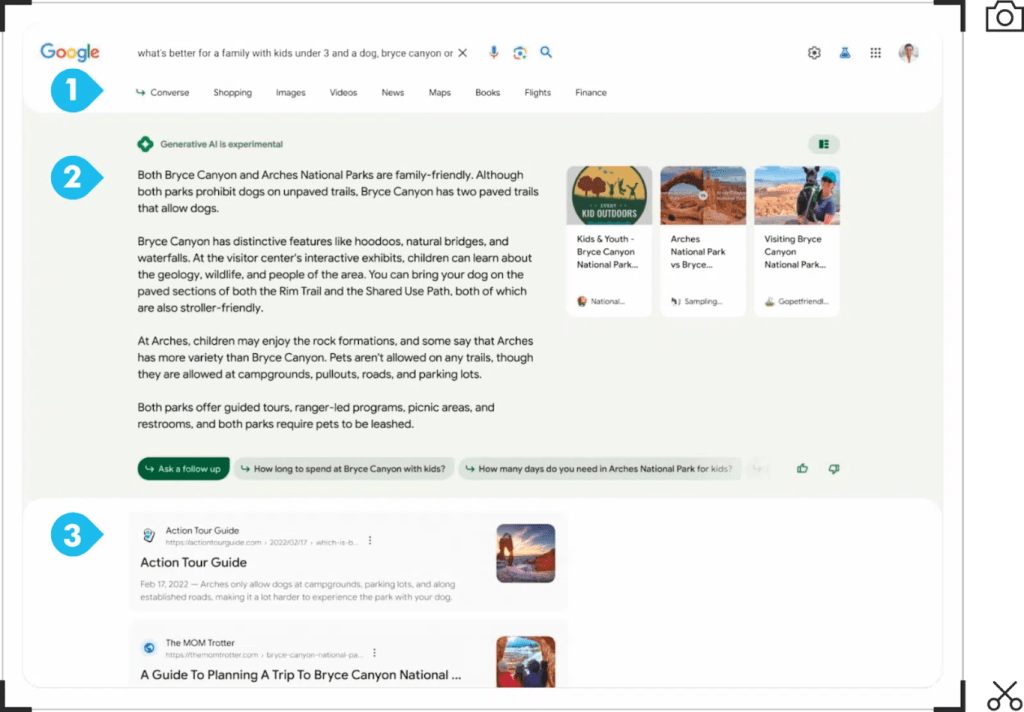
The emergence of SGE is both a promise and a threat to the search industry. Google’s mission to organize the world’s information is taking a new direction, emphasizing user-friendly, context-aware search experiences.
Anticipated Changes:
- Redistribution of the Search Demand Curve: Longer, more context-rich queries will become the norm, altering the traditional search demand curve.
- Changes in CTR Models: The prominence of AI snapshots may reduce CTRs for standard organic results.
- Rank Tracking Complexity: Rank tracking tools will need to adapt to the changing search landscape, potentially incurring higher costs.
- Personalized Results: Context windows and follow-up questions will lead to more personalized search journeys, placing new demands on content creators.
Assessing the Threat
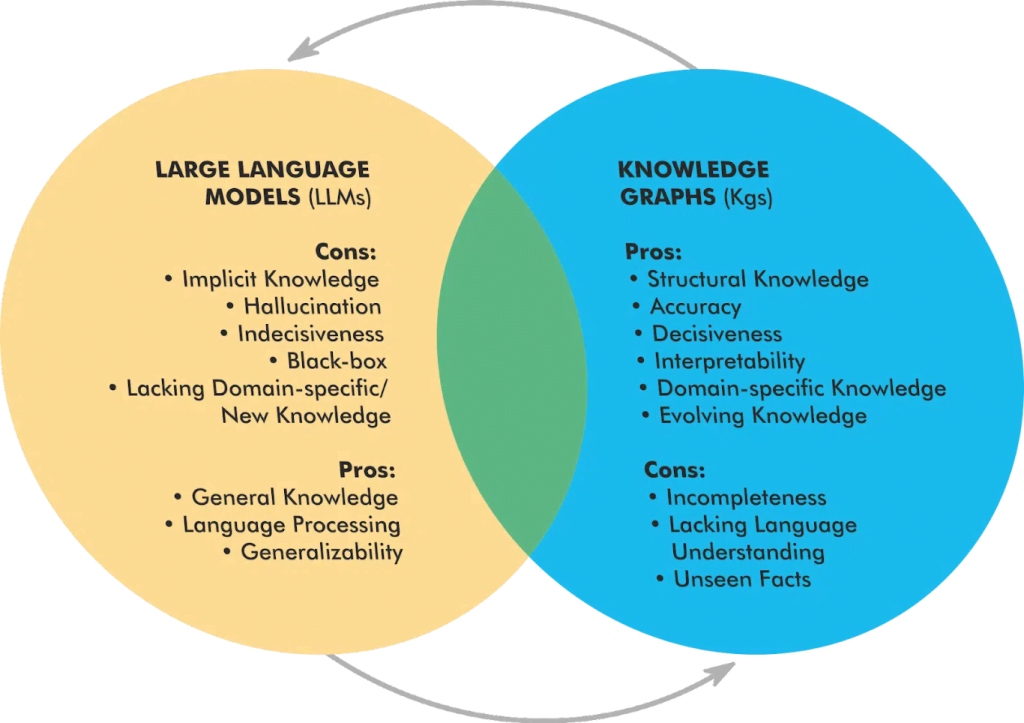
Although data on user behavior in the SGE environment is limited, historical user patterns provide some insights. Users are unlikely to wait for AI snapshots that take more than 14.6 seconds to generate. Additionally, featured snippets historically capture a significant portion of clicks in the SERPs.
Conclusion
Search Generative Experience is poised to redefine online search by making it more user-centric and context-aware. While the transformation presents challenges, it also opens new opportunities for content creators and marketers to adapt and thrive in this evolving landscape. The future of search lies in the delicate balance between AI-driven automation and personalized user experiences.